Uniform variance is a fundamental concept in statistics that plays a crucial role in the reliability and accuracy of data analysis. It's essential for statisticians, data scientists, and researchers to understand this concept to ensure consistent and dependable outcomes in their work. Uniform variance helps maintain stability across datasets, which is vital for making sound decisions and drawing accurate conclusions.
When working with data, it's common to encounter variability, which can affect the accuracy of the results. Uniform variance, or homoscedasticity, addresses this issue by ensuring that the variance within a dataset remains constant across different levels of an independent variable. This consistency allows researchers to make valid inferences and predictions, ultimately leading to more reliable findings.
Incorporating uniform variance into data analysis can greatly enhance the quality of research and decision-making processes. By maintaining a consistent level of variance, researchers can avoid the pitfalls of skewed or biased results. This article will delve into the various aspects of uniform variance, its importance, and how it can be applied to different fields of study. With a comprehensive understanding of this concept, you'll be better equipped to tackle statistical challenges and achieve more accurate results.
Read also:Mastering C Sharp Language From Basics To Advanced Concepts
Table of Contents
- Why is Uniform Variance Important?
- What is Uniform Variance?
- Historical Background of Uniform Variance
- Applications of Uniform Variance
- Benefits of Ensuring Uniform Variance
- Challenges in Maintaining Uniform Variance
- Methods to Test for Uniform Variance
- How to Adjust for Non-Uniform Variance?
- Case Studies Illustrating Uniform Variance
- Tools and Software for Analyzing Uniform Variance
- Educational Resources on Uniform Variance
- Frequently Asked Questions
- Conclusion
Why is Uniform Variance Important?
Uniform variance is a cornerstone of statistical analysis, providing a foundation for reliable and accurate data interpretation. Its importance cannot be overstated, as it ensures that the variability within a dataset does not skew the results or lead to incorrect conclusions. By maintaining a consistent level of variance, researchers can confidently make predictions and draw inferences from their data.
The significance of uniform variance extends across various fields, including economics, psychology, and natural sciences, where accurate data analysis is crucial for informed decision-making. For instance, in economics, understanding uniform variance helps in evaluating market trends and consumer behavior, while in psychology, it aids in assessing the effectiveness of treatments or interventions.
Impact on Statistical Models
Statistical models rely heavily on the assumption of uniform variance to provide accurate results. When this assumption is violated, the models become less reliable, leading to potential errors in predictions and conclusions. By ensuring uniform variance, researchers can trust the outputs of their statistical models and make informed decisions based on these results.
Role in Hypothesis Testing
In hypothesis testing, uniform variance plays a critical role in determining the validity of the test results. It ensures that the test statistics are distributed correctly, allowing researchers to accurately assess the significance of their findings. Without uniform variance, the risk of Type I and Type II errors increases, compromising the integrity of the research.
What is Uniform Variance?
Uniform variance, also known as homoscedasticity, refers to the condition where the variance within a dataset remains constant across different levels of an independent variable. This concept is essential for ensuring the reliability and accuracy of statistical analyses, as it prevents skewed or biased results.
In practical terms, uniform variance implies that the spread of data points around the mean is consistent, regardless of the value of the independent variable. This consistency allows researchers to make valid inferences and predictions, ultimately leading to more reliable findings.
Read also:Effective Ways To Reduce Back Fat A Comprehensive Guide
Comparison with Heteroscedasticity
Heteroscedasticity, on the other hand, occurs when the variance within a dataset is not constant and varies across different levels of an independent variable. This can lead to biased results and incorrect conclusions, as the statistical models may not accurately capture the underlying relationships within the data.
Significance in Regression Analysis
In regression analysis, uniform variance is crucial for ensuring the accuracy of the model's predictions. When the assumption of uniform variance is violated, the regression coefficients may be biased, leading to incorrect inferences and predictions. By maintaining uniform variance, researchers can trust the outputs of their regression models and make informed decisions based on these results.
Historical Background of Uniform Variance
The concept of uniform variance has its roots in the early development of statistical methods and theories. Understanding its historical background provides valuable insights into its significance and evolution in the field of statistics.
Uniform variance emerged as a critical concept during the development of classical statistical techniques, such as linear regression and analysis of variance (ANOVA). These techniques rely on the assumption of uniform variance to provide accurate and reliable results, highlighting its importance in the early days of statistical analysis.
Key Contributions to the Development of Uniform Variance
Several prominent statisticians and researchers have contributed to the development and understanding of uniform variance. Their work has laid the foundation for modern statistical methods and techniques, emphasizing the importance of maintaining consistent variance within datasets.
Evolution of Statistical Methods and Uniform Variance
As statistical methods have evolved, so too has the understanding and application of uniform variance. Advances in computational power and data analysis techniques have allowed researchers to better assess and ensure uniform variance, leading to more accurate and reliable results in various fields of study.
Applications of Uniform Variance
Uniform variance is a vital concept in numerous fields, enabling researchers and professionals to make informed decisions and draw accurate conclusions from their data. Its applications span a wide range of disciplines, highlighting its importance in both theoretical and practical contexts.
Economics and Finance
In economics and finance, uniform variance plays a crucial role in analyzing market trends, assessing risk, and evaluating investment strategies. By ensuring consistent variance, economists and financial analysts can make more accurate predictions and recommendations, ultimately leading to better decision-making.
Psychology and Social Sciences
In the social sciences, uniform variance is essential for evaluating the effectiveness of interventions, treatments, and policies. By maintaining consistent variance, researchers can accurately assess the impact of various factors on human behavior and social outcomes, leading to more effective strategies and solutions.
Natural Sciences and Engineering
In the natural sciences and engineering, uniform variance is important for ensuring the reliability of experimental results and models. By maintaining consistent variance, researchers can trust the accuracy of their findings and make informed decisions based on their data.
Benefits of Ensuring Uniform Variance
Ensuring uniform variance offers numerous benefits, enhancing the quality and reliability of statistical analyses across various fields. By maintaining consistent variance, researchers can avoid biased results and make more accurate predictions and conclusions.
Increased Accuracy and Reliability
Uniform variance enhances the accuracy and reliability of statistical models, allowing researchers to trust the outputs of their analyses. This, in turn, leads to more informed decision-making and better outcomes in various fields of study.
Improved Validity of Research Findings
By ensuring uniform variance, researchers can improve the validity of their findings, reducing the risk of Type I and Type II errors. This enhances the overall quality of research and allows for more accurate inferences and conclusions.
Enhanced Predictive Power
Uniform variance increases the predictive power of statistical models, allowing researchers to make more accurate predictions and recommendations. This is particularly important in fields such as economics, finance, and the natural sciences, where accurate predictions are crucial for informed decision-making.
Challenges in Maintaining Uniform Variance
While uniform variance is a critical concept in statistical analysis, maintaining it can be challenging. Various factors can contribute to non-uniform variance, leading to biased results and incorrect conclusions.
Identifying Non-Uniform Variance
One of the primary challenges in maintaining uniform variance is accurately identifying instances of non-uniform variance. This requires a thorough understanding of the data and the underlying relationships between variables. Advanced statistical techniques and tools can help researchers detect non-uniform variance and address it accordingly.
Addressing Non-Uniform Variance
Once non-uniform variance is identified, researchers must take steps to address it and ensure consistent variance within their datasets. This may involve adjusting the data, using different statistical models, or applying transformations to achieve uniform variance.
Methods to Test for Uniform Variance
Several methods and techniques can be used to test for uniform variance, allowing researchers to assess the consistency of variance within their datasets. These methods help ensure the accuracy and reliability of statistical analyses and models.
Levene's Test
Levene's test is a widely used method for assessing uniform variance, offering a robust and reliable approach to detecting non-uniform variance within datasets. By comparing the variances across different levels of an independent variable, Levene's test can help researchers identify and address instances of non-uniform variance.
Breusch-Pagan Test
The Breusch-Pagan test is another popular method for assessing uniform variance, particularly in the context of regression analysis. By examining the relationship between the residuals and the independent variables, the Breusch-Pagan test can help identify non-uniform variance and guide researchers in addressing it.
White's Test
White's test is a flexible and versatile method for assessing uniform variance, offering an alternative to traditional tests such as Levene's and Breusch-Pagan. By examining the squared residuals and their relationship with the independent variables, White's test can help researchers identify and address non-uniform variance in their datasets.
How to Adjust for Non-Uniform Variance?
When non-uniform variance is identified, researchers must take steps to adjust for it and ensure consistent variance within their datasets. Various methods and techniques can be employed to achieve uniform variance and enhance the reliability of statistical analyses.
Data Transformations
Data transformations, such as logarithmic or square root transformations, can help address non-uniform variance by stabilizing the variance across different levels of an independent variable. These transformations can enhance the accuracy and reliability of statistical models and analyses.
Weighted Least Squares
Weighted least squares is a technique that assigns different weights to observations based on their variance, allowing researchers to account for non-uniform variance and achieve more accurate results. By adjusting the weights, researchers can ensure consistent variance within their datasets and improve the validity of their findings.
Robust Regression
Robust regression is an alternative approach to traditional regression analysis, offering a way to address non-uniform variance and enhance the reliability of statistical models. By minimizing the influence of outliers and extreme values, robust regression can help researchers achieve uniform variance and more accurate predictions.
Case Studies Illustrating Uniform Variance
Case studies provide valuable insights into the practical applications and benefits of uniform variance across various fields. These real-world examples demonstrate the importance of maintaining consistent variance and highlight the impact of uniform variance on research and decision-making.
Case Study 1: Economics and Market Analysis
In this case study, researchers examined the impact of uniform variance on market analysis and investment strategies. By ensuring consistent variance, the researchers were able to make more accurate predictions and recommendations, ultimately leading to better decision-making and improved financial outcomes.
Case Study 2: Psychology and Treatment Evaluation
In this case study, researchers assessed the effectiveness of a psychological treatment by ensuring uniform variance across different groups. By maintaining consistent variance, the researchers were able to accurately evaluate the treatment's impact and make informed recommendations for future interventions.
Case Study 3: Engineering and Experimental Design
In this case study, researchers explored the role of uniform variance in experimental design and engineering. By ensuring consistent variance, the researchers were able to trust the accuracy of their findings and make informed decisions based on their data.
Tools and Software for Analyzing Uniform Variance
Various tools and software programs can assist researchers in analyzing uniform variance and ensuring consistent variance within their datasets. These tools offer advanced capabilities for detecting and addressing non-uniform variance, enhancing the reliability of statistical analyses.
R and Python
R and Python are popular programming languages for statistical analysis, offering a wide range of packages and libraries for analyzing uniform variance. These tools provide advanced capabilities for detecting and addressing non-uniform variance, allowing researchers to achieve more accurate and reliable results.
SPSS and SAS
SPSS and SAS are well-known statistical software programs that offer comprehensive tools for analyzing uniform variance. These programs provide advanced capabilities for detecting and addressing non-uniform variance, allowing researchers to achieve more accurate and reliable results.
Excel and Google Sheets
Excel and Google Sheets offer basic tools for analyzing uniform variance, providing a simple and accessible way for researchers to assess the consistency of variance within their datasets. While not as advanced as specialized programs, these tools can be useful for basic analyses and preliminary assessments.
Educational Resources on Uniform Variance
Numerous educational resources are available for those interested in learning more about uniform variance and its applications. These resources offer valuable insights and guidance for researchers, students, and professionals seeking to enhance their understanding of this critical concept.
Online Courses and Tutorials
Online courses and tutorials provide a convenient and accessible way to learn about uniform variance and its applications. These resources offer in-depth explanations and practical examples, helping learners gain a comprehensive understanding of this important concept.
Books and Publications
Various books and publications offer valuable insights into uniform variance and its applications across different fields. These resources provide detailed explanations and examples, helping readers gain a deeper understanding of this critical concept.
Workshops and Conferences
Workshops and conferences offer opportunities for researchers and professionals to learn about uniform variance and share their knowledge and experiences with others. These events provide valuable networking opportunities and insights into the latest developments and applications of uniform variance.
Frequently Asked Questions
What is the difference between uniform variance and heteroscedasticity?
Uniform variance, or homoscedasticity, refers to the condition where the variance within a dataset remains constant across different levels of an independent variable. Heteroscedasticity, on the other hand, occurs when the variance is not constant and varies across different levels of the independent variable.
Why is uniform variance important in regression analysis?
Uniform variance is important in regression analysis because it ensures the accuracy and reliability of the model's predictions. When the assumption of uniform variance is violated, the regression coefficients may be biased, leading to incorrect inferences and predictions.
How can I test for uniform variance in my dataset?
Several methods can be used to test for uniform variance, including Levene's test, Breusch-Pagan test, and White's test. These methods help assess the consistency of variance within a dataset and guide researchers in addressing any instances of non-uniform variance.
What are the challenges of maintaining uniform variance?
Challenges in maintaining uniform variance include accurately identifying instances of non-uniform variance and addressing them through data transformations, weighted least squares, or robust regression techniques.
What are the benefits of ensuring uniform variance?
Ensuring uniform variance enhances the accuracy and reliability of statistical models, improves the validity of research findings, and increases the predictive power of analyses, ultimately leading to more informed decision-making and better outcomes.
Which software programs can help analyze uniform variance?
Popular software programs for analyzing uniform variance include R, Python, SPSS, SAS, Excel, and Google Sheets. These tools offer advanced capabilities for detecting and addressing non-uniform variance, enhancing the reliability of statistical analyses.
Conclusion
Uniform variance is a critical concept in statistical analysis, playing a vital role in ensuring the reliability and accuracy of data interpretation. By maintaining consistent variance within datasets, researchers can make valid inferences and predictions, ultimately leading to more informed decision-making and better outcomes in various fields of study.
The importance of uniform variance extends across numerous disciplines, including economics, psychology, natural sciences, and engineering. By understanding and applying this concept, researchers can enhance the quality of their analyses and achieve more accurate and reliable results.
As statistical methods and technologies continue to evolve, the significance of uniform variance will only grow, providing a foundation for accurate and reliable data analysis in an increasingly complex and data-driven world.
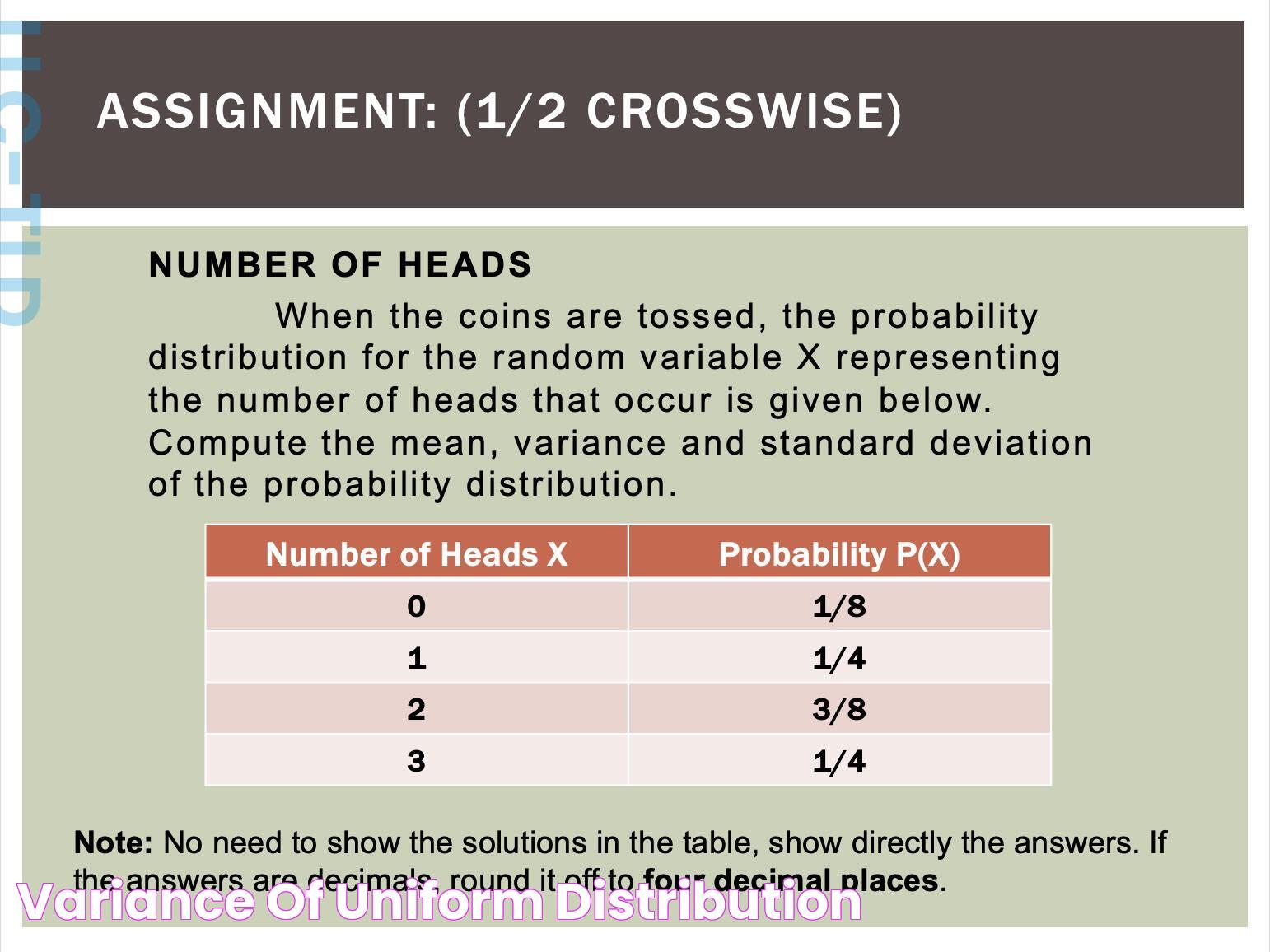
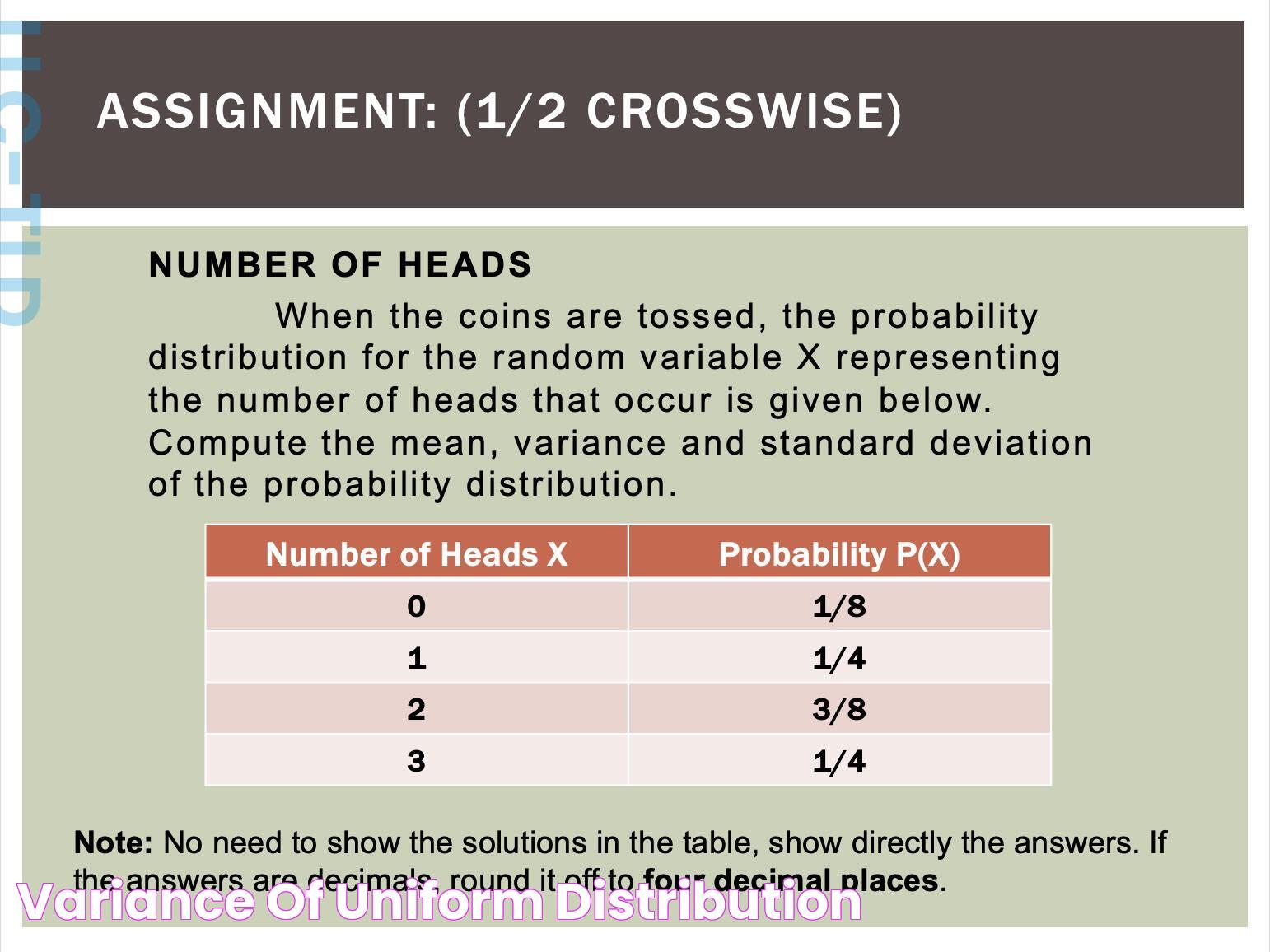